AI models need to be ethical and sustainable. They also need to be community-driven and collaborative in nature. Open source LLMs meet all these criteria and therefore are the future.
Large language models (LLMs) have emerged as a cornerstone in AI evolution. These sophisticated AI models, which process and generate human-like text, are not just technological marvels; they are shaping the future of communication, content creation, and even coding. As organisations and individuals navigate this new landscape, one critical decision stands out — choosing between proprietary and open source LLMs. Let’s delve into the compelling reasons to consider open source LLMs, underscoring the potential risks of overlooking them.
Understanding open source LLMs
Before delving into the intricacies of open source LLMs, it’s essential to understand their foundation. LLMs are a subset of what’s known as foundation models. These are expansive AI models trained on vast amounts of diverse, unlabelled data in a self-supervised manner. The ‘large’ in LLMs isn’t just hyperbole—it reflects the immense scale of data they’re trained on, often reaching petabytes, which translates into a staggering quantity of words and information.
At the heart of LLMs are three core components.
- Data: This is the raw material of LLMs—the vast, unstructured textual data they’re trained on. While a gigabyte of text data might contain roughly 125 million words, LLMs go much further, being trained on exponentially larger datasets.
- Architecture: This refers to the underlying structure of the model. For instance, GPT-3.5 utilises a transformer architecture, which is particularly adept at handling the complexities of natural language due to its ability to process sequences of data and capture contextual relationships within text.
- Training method: The process involves iterative learning, where the model, using its architecture, learns to predict and generate text based on the input data it’s fed. This training is computationally intensive, requiring significant processing power and, consequently, financial resources.
Building and even fine-tuning LLMs demand considerable computational resources, making them costly ventures. Recognising this, certain organisations have stepped forward to bear the financial burden, training these sophisticated models and then releasing them to the open source community. Their contributions ensure that the benefits of LLMs can be accessed and further developed by researchers, developers, and innovators worldwide, fostering a collaborative ecosystem of AI advancement.
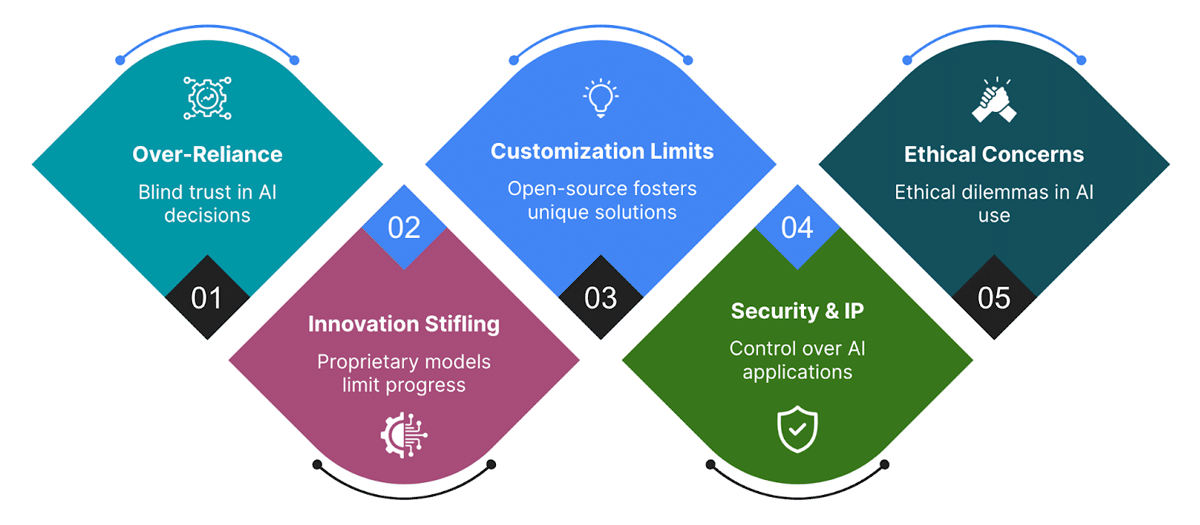
The philosophy behind open source LLMs
The essence of open source LLMs lies in the belief that technological advancements should be a collaborative, shared resource. Organisations like Meta have pioneered this movement, training and releasing state-of-the-art models like LLaMA 2 and the upcoming LLaMA 3. These models, while free to use, are built upon the significant financial investments of their parent companies, reflecting a commitment to open source principles that transcend traditional business models.
Two examples of pioneering open source LLMs are:
- LLaMA series by Meta:
A testament to Meta’s investment in the open source community, these models serve as robust platforms for further innovation. They enable creators to develop solutions that are as diverse as the use cases they envision—from enhancing conversational AI to creating new forms of digital interaction. - Falcon models by the Technology Innovation Institute: The Falcon models embody the practical application of open source philosophy, offering efficiency and a wide knowledge base, thanks to their training on extensive datasets like RefinedWeb. They exemplify how open source can bridge the gap between cutting-edge research and real-world application.
In this context, ‘open source’ signifies more than code; it’s a commitment to collective progress in AI. It represents a future where the most advanced tools in natural language processing are not the exclusive domain of the few but a shared asset for the many, driving forward the boundaries of what we can achieve with technology.
Potential risks of ignoring open source LLMs
Over-reliance on proprietary models
The convenience of proprietary LLMs comes with a hidden cost: over-reliance. This dependency is not just about the technological aspects but extends to decision-making processes where the opacity of these models can lead to unquestioned acceptance of their outputs. Stanford University’s research into AI over-reliance, particularly in high-stakes fields like healthcare and law, underscores the dangers of accepting AI decisions without scrutiny. The study advocates for simpler AI explanations and more accountable decision-making processes, highlighting the need for tools that users can understand and trust. This scenario exemplifies the risks associated with proprietary systems’ lack of transparency, emphasising the importance of open source models that offer greater inspectability and modifiability.
The innovation and sustainability bottleneck
Exclusively focusing on proprietary models not only stifles innovation by limiting access to underlying technologies but also raises significant environmental concerns. The development of open source LLMs fosters a culture of collaborative innovation, where a diverse community contributes to advancements and solutions. This approach not only accelerates technological progress but also distributes the developmental load, reducing the carbon footprint associated with creating large models from the ground up.
Customisation and IP concerns
Before going into the depth of risks around customisation and IP, let’s try to understand the need with a scenario. In the world of generative AI applications, the debate often centres on whether to develop custom solutions for specific use cases. Use cases are endless, which means creation of custom applications for every use case may lead to a huge number of applications running around the organisation. Can we then take an approach that OpenAI took? They provided a chatbox and said, do whatever you want to do! We all then learnt different prompting techniques to use this in our world. While connections to structured databases or external knowledge bases may necessitate unique applications, many needs can be met through the adept use of existing LLMs as is. The crux lies in crafting effective prompts to leverage the model’s inherent knowledge base for tasks like generating organisation-specific documents or marketing content.
However, apprehensions about intellectual property (IP) and data privacy significantly deter organisations from utilising platforms like ChatGPT, even in its enterprise variants. The reluctance stems from concerns over data security and IP confidentiality, despite assurances from service providers about data protection. This scenario underscores a critical advantage of open source LLMs: the ability to host these models within an organisation’s own infrastructure, offering complete control over data and IP without compromising on the capabilities offered by generative AI.
Imagine creating a marketing campaign or drafting emails with a tool that mirrors ChatGPT’s functionality but operates entirely within your organisational boundary, disconnected from the internet, thus safeguarding your data. This vision is not just feasible but increasingly necessary in a landscape where data privacy and security are paramount. Open source LLMs offer a tangible solution to these challenges, providing the benefits of advanced AI applications while ensuring data remains within the protective perimeter of the organisation.
Misuse and ethical dilemmas
AI’s capability to generate convincing fake content or to be used in scams, such as voice cloning scams, poses significant ethical and security risks. These technologies have been used to fraudulently impersonate individuals, demonstrating the consequences of AI misuse. Furthermore, instances of AI involvement in creating ransomware or legal disputes over practising law without a licence illustrate the legal and ethical quagmires arising from deploying AI without adequate oversight.
We can take control of the guardrails to be put at the top of the OS LLMs we have deployed.
- Unauthorised data scraping: This involves collecting data from various sources without permission, which can lead to privacy violations. For instance, there have been cases where large datasets were used to train AI models without the explicit consent of the individuals whose data was included. Imagine a guardrail that sanitises your data that the LLM is consuming.
- Creation of deepfakes: Deepfake technology uses AI to create highly realistic but entirely fabricated images, videos, or audio recordings. This can be used maliciously to impersonate individuals, spread misinformation, or commit fraud. Imagine a guardrail that takes care of unauthorised creation of such outputs.
- AI-generated misinformation: An example of this is when AI, like ChatGPT, generates false information, such as incorrectly implicating individuals in legal cases. This unpredictability of AI-generated content can have serious implications, causing harm to reputations or even leading to legal action. Imagine a guardrail that includes citations for every response.
These incidents underscore the complexity and potential risks associated with deploying AI technologies, especially when they are not transparent or open to scrutiny. Proprietary models, which are closed source and controlled by specific organisations, often lack the transparency that allows external parties to audit or understand their decision-making processes fully. This opacity can exacerbate data privacy and security risks, making it challenging to ensure the ethical and secure use of AI.
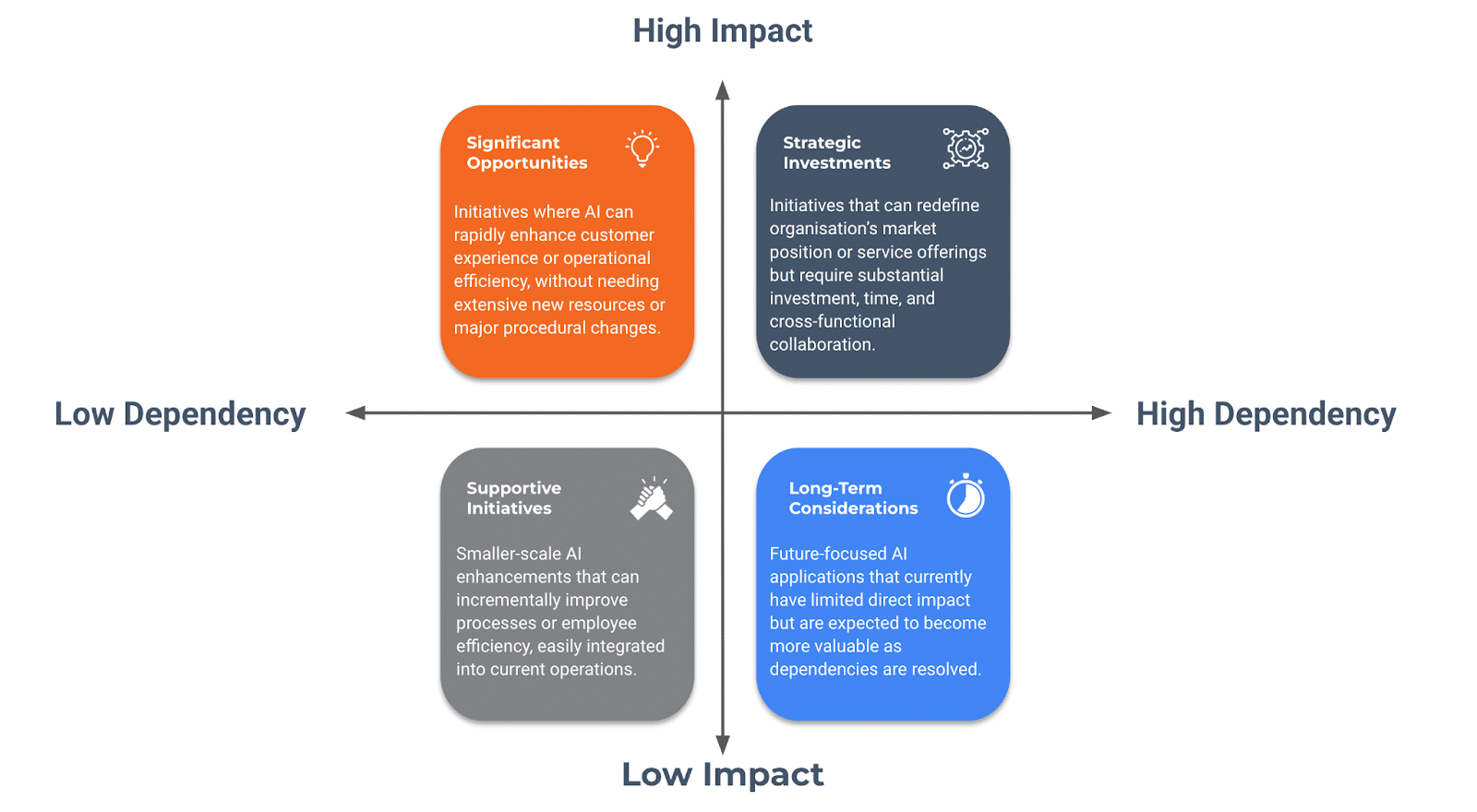
Sustainable AI: A greener path with open source LLMs
Before we dive into recommendations, it’s crucial to address our responsibility for sustainable innovation. It’s about ensuring that the advancements we celebrate today do not compromise the very planet we call home. As we stand on the cusp of these technological leaps, we must anchor ourselves with a commitment to sustainability.
Harnessing foundation models for sustainable advancement: In the pursuit of innovation, open source LLMs such as LLaMA and Falcon models represent a paradigm shift towards sustainability. These foundation models provide a springboard that drastically cuts down the cost and complexity of crafting a language model from the ground up. This strategy isn’t just economically savvy; it also aligns with eco-friendly practices by reducing the computational firepower and energy consumption typically associated with training models from zero, advocating for a greener AI future.
The green imperative: The carbon output of training a single advanced AI language model can parallel the lifetime emissions of multiple vehicles. This stark environmental cost stems from the energy-intense nature of the training process, which involves vast datasets and intricate calculations.
Initiatives to steer AI towards a more sustainable trajectory encompass transparent carbon footprint accounting for AI, adoption of green energy sources, strategic management of computational resources, advancement in hardware efficiency, and the strategic selection of models suited for specific tasks. For instance, training the BLOOM model by Hugging Face on a supercomputer predominantly powered by nuclear energy resulted in a notably lesser environmental impact compared to the creation of models like GPT-3.
Ongoing research into deep learning efficiency is also pivotal. Innovations include neural architecture search, which aims to design leaner models, and the ‘lottery ticket hypothesis’, which suggests that training a small yet potent subset of a model could lead to significant reductions in energy use.
By leveraging the groundwork laid by existing open source LLMs, organisations can stride towards their AI goals with a conscientious approach towards the environment, aligning cutting-edge technology with the pressing need for sustainability.
Future of open source LLMs and their impact
Diverse ecosystem of models
The AI industry is witnessing a significant shift towards a more decentralised approach to the development of large language models (LLMs), with entities like Meta pioneering this movement. This trend underscores a collective effort to prevent the monopolisation of generative AI technologies, ensuring a broad range of stakeholders can contribute to and benefit from these advancements. The emergence of open source, commercially usable LLMs enables organisations to implement custom solutions tailored to their unique requirements, fostering a rich ecosystem of AI tools.
Innovation through experimentation
Models like TinyLLaMA and Gemini Nano exemplify the industry’s move towards smaller, more efficient LLMs capable of running on devices with limited computing resources. This innovation opens up new avenues for experimentation, allowing developers and organisations to explore AI functionalities natively on mobile devices, enhancing user experiences and operational efficiencies.
By focusing on models that can be deployed on lower compute devices, developers can craft tailored AI solutions that maintain high levels of performance and security, even within the constraints of mobile technology.
Community-driven innovation
I am a huge supporter of community lead tech and in my opinion this is going to be the driving factor in future.
The growing open source AI landscape, significantly propelled by major tech entities like Meta, is testament to the pivotal role the community plays in the evolution of AI. These contributions are not just about offering tools; they’re about nurturing an ecosystem where innovation, creativity, and collaboration intersect to push the boundaries of what AI can achieve.
The impact of open source contributions
Meta’s decision to make these advanced LLMs accessible has catalysed a wave of innovation within the AI community. This openness allows creators, developers, and researchers to experiment, modify, and implement these models in myriad ways, leading to the development of novel applications and solutions. Such a collaborative environment accelerates the pace of AI innovation, ensuring that advancements are not siloed within the confines of large corporations but are shared, improved upon, and democratised.
The role of community in AI evolution
The active participation of the open source community in AI development is crucial. It brings together diverse perspectives and expertise, fostering a rich breeding ground for innovative ideas and approaches. Platforms and frameworks like LangChain and Ollama, which emerge from this collaborative effort, exemplify the dynamic nature of the community’s contribution to AI. They provide the scaffolding for integrating LLMs into practical applications, further expanding the utility and reach of AI technologies.
The open source model, championed by entities releasing these large language, voice, and vision models, ensures that the power of AI is not confined to a select few but is available to a broad spectrum of innovators across the globe.
Future horizons
As the open source AI community continues to grow, fuelled by the contributions from major tech companies and the relentless creativity of individuals around the world, we stand on the brink of a new era in AI. This era will be characterised by an explosion of AI-powered applications and services, tailored to meet the specific needs and challenges of different sectors and societies. The community’s role in this cannot be overstated.
Recommendations for organisations
To leverage open source LLMs effectively, organisations should consider a structured and detailed approach that encompasses evaluation, customisation, and a focus on data privacy and security. Here’s a synthesis of the latest recommendations for organisations considering open source LLMs, based on insights from recent articles.
Evaluating and selecting open source LLMs
Organisations should start by evaluating the capabilities and performance of various open source LLMs to ensure they meet specific needs. Popular models like LLaMA 2, BLOOM, and Falcon-180B offer a range of capabilities from multilingual support to high performance in natural language processing tasks. It’s crucial to choose an LLM that not only aligns with your organisational requirements but also offers the flexibility for customisation and fine tuning.
Customisation and integration
The open source nature of these models allows for significant customisation. For instance, Meta’s LLaMA 2 and Salesforce’s XGen-7B demonstrate the potential for customisation in coding and long-context tasks. Integrating these models into existing systems and workflows efficiently requires developing custom APIs or connectors, ensuring a seamless transition, and maximising the utility of these AI tools.
Advanced data privacy and security measures
A paramount advantage of open source LLMs is the capability for local, on-premise deployment, which is critical for maintaining data security and privacy.
- Localised data processing: Emphasise the development and use of open source tools that enable localised data processing, reducing the reliance on cloud-based services and enhancing data privacy and security.
- Comprehensive security protocols: Implement comprehensive security protocols that go beyond data anonymisation, such as end-to-end encryption for data in transit and at rest, regular security audits, and vulnerability assessments.
Sustainable and scalable adoption
For sustainable and scalable adoption, organisations should focus on:
- Training and fine-tuning: Invest in training and fine tuning the chosen LLM with your organisation’s specific data, aiming to align its outputs closely with your business objectives. This could involve leveraging domain-specific datasets to enhance the model’s relevance and accuracy for your applications.
- Ongoing monitoring and maintenance: Establish processes for regular performance monitoring, maintenance, and updates to the LLM. This includes keeping abreast of the latest developments in open source LLMs and ensuring that the model continues to meet your needs efficiently.
- Community engagement and contribution: Engage with the broader open source community to stay informed about the latest advancements and contribute to the development of these technologies. Participating in this ecosystem can provide insights into best practices, emerging trends, and potential collaborations.
Striking the balance: Innovation and ROI in implementing open source LLMs
Reflecting on my journey with clients exploring the transformative potential of generative AI—spanning large language models, voice, and vision technologies—I have come to recognise the critical importance of striking a delicate balance between innovation and tangible returns on investment (ROI). It’s a dynamic that requires a nuanced approach, balancing the allure of wide-ranging automation possibilities with the pragmatic need for strategic, sustainable deployment.
When clients are introduced to the capabilities of generative AI, their enthusiasm often translates into a vast array of potential use cases. Yet, amidst this enthusiasm, the challenge lies in discerning which use cases to prioritise for implementation. The selection process depends on identifying where generative AI can make the most immediate and impactful difference in streamlining workflows today.
Adopting open source LLMs within an organisation’s ecosystem necessitates a considered approach. Even the integration of a single AI solution can significantly disrupt existing workflows, necessitating a period of adjustment and synchronisation. During this phase, workflows might need to be modified to accommodate the AI solution, and vice versa. However, with patience and strategic planning, this period of adjustment leads to a stable integration, where the benefits of the AI solution become clear and measurable.
The key, from my perspective, is maintaining a clear vision of both the short-term and long-term impacts of these innovations. Innovation cannot be bracketed into a one-size-fits-all approach. Instead, it requires a commitment to an iterative development process, where quick wins are celebrated as milestones towards longer-term transformational goals. This approach not only ensures the sustainability of the AI initiative but also keeps the focus firmly on the business and revenue implications. A quick matrix that I started using to understand from organisations what would make sense to strike the balance is shown in Figure 2.
In discussions with clients, I emphasise the importance of this balanced approach. It’s crucial to select use cases that not only promise to disrupt and improve current workflows but also align with the organisation’s strategic objectives and capacity for change. By doing so, we can navigate the initial disruption to achieve a harmonious integration of AI technologies, ultimately realising their substantial ROI.
As I look forward, the journey with open source LLMs is far from complete. The key lies in maintaining a balance—between exploring new frontiers of technology and grounding these explorations in strategic, sustainable practices that yield real value. This balance is not just a goal but a guiding principle for navigating the future of AI, ensuring that as we move forward, we do so with purpose, foresight, and a commitment to leveraging technology in ways that are transformative, ethical, and ultimately, beneficial for all.