“The integration of AI on the cloud and AI at the edge is of utmost importance in today’s technological landscape,” says Ajay Chaudhary, Associate Vice President – Engineering, GlobalLogic.
From automobiles to industrial equipment, everything is becoming smart. And it is becoming increasingly clear that the entire demand for ‘smartness’ cannot be met by cloud computing alone. As the number of users increases exponentially, it takes a toll on cloud computing infrastructure and processing delays creep in. To state the obvious, latency is something we cannot live with. In a self-driving car, for instance, a microsecond’s delay could cause an accident. Moreover, can smart devices afford to become dumb when they lose connectivity? Say, when a surveillance drone flies into a deep forest where there is no mobile connectivity.
It is becoming increasingly clear that the traditional modus operandi, in which edge devices simply sense and actuate, while the entire processing and decision-making is done on the cloud, will not suffice in the future. The tech world is transitioning to hybrid processing – where part of the processing happens at the edge, and the rest on the cloud. And finally, with the rise of AI, edge computing is getting the limelight it deserves. In fact, at this year’s Mobile World Congress some companies demonstrated the capability of edge devices to run even large language models (LLMs), and it is believed that this will soon become the norm.
In a conversation with Janani G. Vikram, Ajay Chaudhary, Associate Vice President – Engineering, GlobalLogic, speaks about recent tech developments in edge computing, 5G’s helping hand, AI at the edge, and much more.
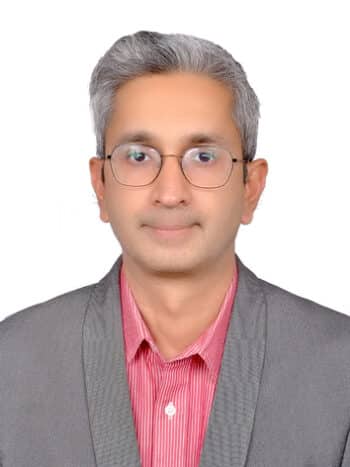
Q. Can you tell us about some of the recent developments in edge computing?
A. There have been significant advances in edge computing. Many of the leading tech giants have brought in hardware architectural innovations, which provide increased computation, storage, and overall processing at the edge. Some of the hardware development boards are increasingly accessible from a cost perspective. Also, better connectivity solutions enabled by 5G bring faster communications between edge devices and enterprise systems.
We can see many segments benefitting from these recent advancements…
- Drones: Drones heavily rely on edge computing architectures, with an increasing amount of storage capacity that enables them to store business algorithms for situation-based decision-making. In the past, drones simply acted on commands received from remote control rooms. However, with their new decision-making capabilities, drones have become invaluable in remote locations where communication is limited. For instance, agricultural drones equipped with pesticide sprinklers can take videos and analyse local conditions of insect infections, allowing them to decide precisely where to sprinkle pesticides. This intelligent approach saves cost, and effort, and reduces pesticide wastage, as the pesticide is targeted only to the affected areas. Furthermore, modern edge devices have larger storage capacities, allowing drones used in military surveillance to store more data for longer durations. This improved endurance enhances their effectiveness in extended operations.
- Augmented reality (AR)/ Virtual reality (VR)/Mixed reality (MR) based field operations: Field operations are inherently complex due to remote locations and constrained resources. Elevator maintenance serves as a challenging example. Elevators come in various models, making it difficult for a technician to be an expert in all of them. However, in such scenarios, field technicians can receive support through AR/VR-based devices, which enable them to access large digital models, manuals, and an extensive knowledge database. By utilizing these AR/VR devices, technicians can quickly find assistance even in remote operational sites, such as basements where connectivity is limited.
- Remote diagnostics: Remote diagnostics kits are gaining popularity as they monitor the real-time status of machines, allowing for prompt diagnosis and error rectification as needed. Advancements in edge computing facilitate closer collaboration with the command centre through video-based assistance, enabling quick issue resolution and avoiding any disruptions in operations.
Q. How will 5G further fuel the growth of edge computing?
A. 5G provides an average data transfer speed that is ten times faster than 4G, making it a key enabler of edge-based systems. This enhanced connectivity enables edge devices to communicate more quickly with enterprise systems, resulting in faster decision-making based on the data collected at the edge.
For instance, telemedicine consultations become more effective as patient X-rays can be sent rapidly to a centralized hospital for analysis, and diagnoses can be delivered on a near real-time basis. There are numerous other use cases where seamless connectivity between edge devices and enterprise systems is essential, and all these use cases can greatly benefit from the utilization of 5G.
Q. Amongst the emerging applications of edge computing, which ones do you consider the most important?
A. In the ever-evolving landscape of technology, edge computing has emerged as a transformative force, enabling real-time data processing and decision-making at the edge of networks. Amongst the emerging applications of edge computing, the most important ones include manufacturing, smart cities, healthcare, as well as autonomous vehicles and military operations:
- Industry 4.0 implementation: Industry 4.0 provides automation of manufacturing processes. During the manufacturing process, certain autonomous processes require real-time decision-making to avoid any disruption in the production line. Edge devices are responsible for controlling these processes. For example, a car assembly line uses a device with a camera to track different parts and raise a notification when any component is about to run out near the assembly line stocks, thus preventing any disruption in the car assembly process.
- Smart city implementations: Smart city requires real-time data coordination between different city-based systems like intelligent traffic systems, intelligent lighting systems, and smart grids, to name a few. Traffic lights require edge devices to operate traffic lights in a round robin fashion. Similarly, street lights are connected to edge devices to get commands like when to switch on or off.
- Medical IoT implementations: Medical devices like electrocardiogram (ECG) monitors have edge devices that store business rules and send alerts to medical professionals when patient vitals are not normal. This provides real-time status of patients, to plan the medical procedures if needed. There is surgical equipment that requires mandatory sterilization after every procedure. Edge devices installed in such medical devices alert for required sterilization that is monitored using the opening of the surgical kit.
- Autonomous vehicle implementations: Autonomous vehicles possess edge devices where they store different AI models with details of different traffic conditions and behaviour of the car. Based on the traffic conditions, the car follows the command from the edge device and automatically gets driven accordingly.
- Military operations: Edge computing devices are the main components of military equipment. Edge devices have business rules for different battle conditions. These edge devices acquire data in real time and respond as per the battlefield conditions.
Q. It is clear that AI on the cloud is not enough, and the industry must focus on AI at the edge – what are your closing comments on this?
A. The integration of AI on the cloud and AI at the edge is of utmost importance in today’s technological landscape. Cloud-based AI has revolutionized data processing and analysis with its vast computational capabilities and storage. However, edge AI complements this approach by offering unique advantages. It involves deploying AI algorithms and models directly on edge devices or gateways, closer to the data source, providing reduced latency, enhanced privacy and security, offline functionality, and bandwidth optimization.
By harnessing the strengths of both cloud-based AI and edge AI, industries can create a comprehensive and efficient AI ecosystem that addresses diverse use cases and ensures real-time decision-making and seamless operations. The convergence of these two approaches paves the way for a dynamic future of AI applications, driving innovation and progress across various industries, including autonomous driving, medical diagnostics, and military operations, where edge AI plays a pivotal role in instant decision-making and adaptability to changing scenarios.