As AI evolves, we must navigate the ethical and practical challenges it poses. Here’s a summary of key AI trends and their significance in shaping the future of industries.
In the field of artificial intelligence, there is a common misconception that artificial intelligence (AI), machine learning (ML), deep learning (DL), genetic algorithms and neural networks are synonymous or do the same function but are merely matured by algorithms.
Actually, artificial intelligence (AI) is the traditional model devised to mimic human brain functions by computers using a technology-driven approach and mathematical and statistical models (e.g., probability). Later, machine learning (ML) was conceived to make programming models of brain functions and improve the behaviour of AI models through various learning approaches, storing the results of machine learning models for future predictions. This was inspired by the use of the neural network, which is the core storage layer of the human brain. To improve the processing efficiency of this neural network-based solution, a distributed model was implemented for faster processing and simulation of various brain functions like object detection and analytical abilities.
Genetic algorithms improve deep learning models with self-learning ability and intuitiveness to maximise the application of AIML models, reduce the effort in training them, and improve the knowledge base in neural networks. Generative AI, for example, uses large language models (LLM) to handle activities like content summarisation, content regeneration, and content extraction through self-learning capabilities. All these methods contribute to improving the ability of computer-based applications to emulate human-like thinking, reducing the effort in building and training the models, and cutting costs in time-to-market, with the flexibility to apply them in multi-domain solutions.
Importance of tracking AI trends
The importance of tracking AI trends can be summarised in the following points.
Stay ahead of the competition: AI is rapidly evolving, and businesses aiming to remain competitive need to be aware of the latest trends. By tracking AI trends, businesses can identify new opportunities and threats, and develop strategies to stay ahead.
Identify new market opportunities: AI is being used in a wide variety of industries, with new applications emerging all the time. Tracking AI trends helps businesses identify untapped market opportunities.
Make better strategic decisions: AI can be used to collect and analyse data, identify patterns, and make predictions. Businesses can use this information to make better strategic decisions about their products, services, and operations.
Improve efficiency and productivity: AI can automate tasks, improve decision-making, and optimise processes. By keeping an eye on AI trends, businesses can find ways to improve their efficiency and productivity.
Reduce costs: AI can automate tasks currently performed by humans, resulting in cost savings. Tracking AI trends enables businesses to identify cost-saving opportunities.
Improve customer experience: AI can personalise customer experiences, provide better customer service, and predict customer needs. Tracking AI trends helps businesses improve their customer experience.
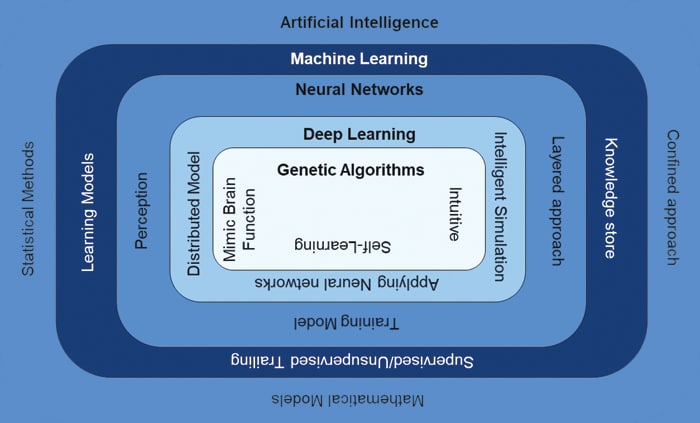
Explainable AI
Explainable artificial intelligence (XAI) refers to the development of AI systems that can provide understandable and transparent explanations for their decision-making processes. XAI is essential because traditional AI models, like deep neural networks, often operate as ‘black boxes’, making it challenging to comprehend why they reach specific conclusions or predictions.
XAI aims to bridge this gap by enabling humans to understand and trust AI systems. It involves techniques such as model interpretability, feature importance analysis, and rule-based systems to make AI more transparent. This transparency enhances accountability, aids in debugging and improving models, and ensures that AI systems adhere to ethical and regulatory standards.
Various approaches to XAI exist, with the best approach depending on the specific AI model and application.
Feature importance: Identifies the most important features for an AI model’s decision-making
Counterfactual explanations: Shows how an AI model’s prediction would change if input features were different
Local explanations: Explains an AI model’s decision for a specific input example
Model introspection: Allows users to explore an AI model’s internal workings
XAI is a rapidly growing field with ongoing research, and has the potential to make AI systems more transparent, trustworthy, and fair.
As an example, a hospital can use XAI to explain how a machine learning model is making decisions about cancer treatment. This can help doctors to understand the model’s reasoning and to make better treatment decisions. XAI can also be used to identify biases in the data or the model, which can help to improve the accuracy and fairness of the model’s predictions.
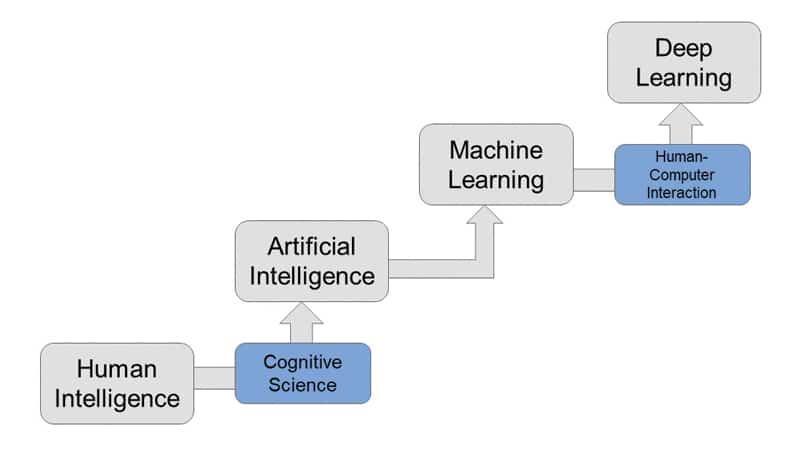
Generative AI
Generative AI is at the forefront of creative innovation. Through neural networks, this technology enables the generation of content, art, music, and even human-like text. Artists, designers, and authors are using generative AI to create one-of-a-kind and inventive works. AI-powered technologies aid in the creation of unique designs in the field of design, while generative AI composes tunes that fascinate listeners in the field of music. However, ethical concerns arise due to its potential for generating deepfake content and misinformation.
In recent years, generative AI has made considerable advances, allowing it to create highly realistic and imaginative content across a wide range of areas. The invention of generative adversarial networks (GANs), a type of machine learning model, is one of the most breakthrough advances in generative AI. GANs are made up of two neural networks: a generator and a discriminator. The generator generates new data instances, while the discriminator validates them. These two networks compete in a process in which the generator wants to create data that is indistinguishable from genuine data and the discriminator aims to correctly categorise real and generated data. The following are some of the most recent advances in Generative AI.
High-quality image synthesis: GANs can generate high-resolution images that often resemble real photographs. This can be used to create art, design, and even photorealistic representations of non-existent things or landscapes.
Style transfer and artistic rendering: Generative AI allows users to apply one image’s style to another’’s content. AI-generated art has emerged as a result, combining the qualities of many styles and materials.
Text-to-image synthesis: Generative AI can now create visuals that closely match the specified scenarios when given a textual cue, making it useful in sectors such as creative writing and advertising.
Video generation and manipulation: Advances in GANs simplify video generation and manipulation, enabling AI to produce new video content, alter current video footage, and even interpolate between frames to produce seamless transitions.
Voice and audio synthesis: Beyond images, Generative AI expands into audio synthesis, creating lifelike speech, music, and sound effects for entertainment, gaming, and voice assistants.
Drug discovery and molecule generation: Generative AI aids in identifying novel medicinal compounds by producing chemical structures that match specified criteria. This speeds up the drug discovery process by allowing researchers to investigate a broader range of possible molecules.
Realistic human face generation: AI models create incredibly convincing human faces, raising ethical issues about future applications in deepfake content creation.
AI-generated content curation: Generative AI supports content creators by suggesting ideas, generating drafts, and even composing music or text segments depending on specific topics or inputs.
Cross-domain applications: Generative AI finds applications across domains, allowing artists, designers, scientists, and engineers to use its creative powers in a variety of applications.
As generative AI advances, it has the potential to transform businesses, ignite creativity, and provide new ways to engage with and produce material. However, ethical concerns, such as the possibility of misuse and the need to ensure the responsible use of AI-generated content, must be addressed as this technology advances.
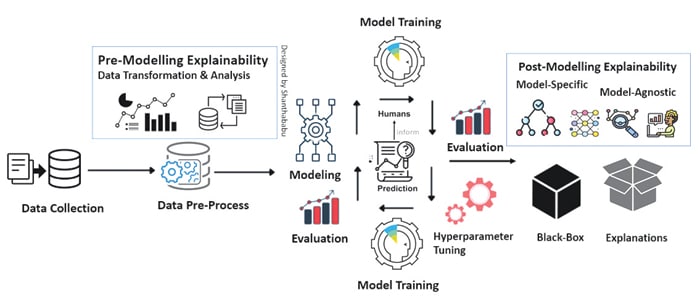
AI fairness and bias
Famous AI guru Ray Kurzweil coined the term ‘singularity’ in artificial intelligence, signifying the convergence of AI and human intelligence or making AIML accuracy approach human-like thinking. To achieve this highest level of accuracy in ML training, modelling, and functionality, it is of the utmost importance to address fairness and bias in AIML implementation.
Efforts to reduce or avoid bias in developing an AIML solution across various stages naturally lead to fairness in execution and results. Bias cannot occur on its own in the AIML stages by the system, but can result from human inputs.
For example, when preparing data for ML model training, one should have fairly distributed, unbiased data to prevent biased outcomes. Similarly, when labelling and grouping the data, training the model to simulate human-like thinking, deploying the model with knowledge gained from training, and interpreting the results from ML execution, it is essential to avoid pre-judgement or biased personal interests that could compromise fairness in building the entire system.
This includes avoiding race, income category, sexual orientation, gender, religion, marginalisation of the user and any discrimination of the user data when preparing the training data, as well as training the system and interpreting the results from the machine learning execution.
Various tools like IBM AI Fairness 360, Microsoft Fairlearn, and Google What-if are invaluable for validating and identifying if the training model and data collection has any bias in preparation and implementing fairness in the AI applications.
AI governance
AI governance is a set of principles, policies, and practices that organisations use to manage the development, deployment, and use of AI systems. It is important to have AI governance in place to ensure that AI systems are used in a responsible and ethical way while mitigating the risks associated with AI.
Some of the key aspects of AI governance are:
Data governance: Managing data used for training and deploying AI systems to ensure that the data is accurate, complete, and unbiased.
Algorithmic accountability: Ensuring that AI systems are transparent and explainable. It is important to be able to understand how AI systems make decisions to identify and address any biases or unfair outcomes.
Human oversight: Ensuring that humans are involved in the development and deployment of AI systems so that these systems are used in a safe and responsible way.
Risk management: Identifying and mitigating the risks associated with AI systems including bias, discrimination, and job displacement.
Evolution of AI trends in the next five years
Artificial intelligence (AI) is a rapidly evolving field, and several trends are expected to shape the industry in the next five years. Here are some upcoming AI trends.
- Reinforcement learning and self-learning systems: These systems are expected to become more prevalent, capable of learning from their own experiences and improving their performance over time without human intervention.
- Autonomous vehicles: AI is a crucial component of autonomous vehicles, enabling them to perceive their surroundings and make decisions.
- Generative AI: The use of generative AI to create new content, such as images, videos, and music is expected to expand across various industries, including entertainment and advertising.
- Digital twinning: Creating digital replicas of physical objects or systems is set to become more prevalent in industries, including manufacturing and construction.
- Federated learning: This is a distributed machine learning approach that allows AI models to be trained on data that remains on users’ devices. This can help to address privacy concerns and improve the security of AI systems.
- Multimodal learning: AI models can learn from multiple data types, such as text, images, and audio. This can help to improve AI system accuracy and robustness.
These trends are expected to have a significant impact on various industries, including healthcare, transportation, cybersecurity, and entertainment. As the field of AI evolves, these trends will shape the future of many industries.