The 21st century has seen the rise of machine learning (ML) and artificial intelligence (AI). Many mundane tasks are today done by machines. ML and AI have pervaded all aspects of human life, without exception. So will machines soon overtake us in an Orwellian manner? Read on for some insights into this worrying question.
Data is the currency for the new age, particularly with the advent of machine learning. From Google to Tencent, companies are locked in a battle to be the first to create and combine the most efficient models in an attempt to create artificial general intelligence. As businesses scramble to build the best products, they have created a wave of artificial intelligence (AI) that has swept the world off its feet. AI has become more than just a buzzword as technologies have emerged that are capable of changing the world, though people are still unclear about whether this will be for the better or worse. What we do know, however, is that a revolution is under way and it will leave nothing untouched.
From social media to kitchen faucets, companies are targeting the most mundane of products to incorporate some semblance of human intelligence to purportedly make life easier for us.
We have artificial intelligence embedded the in core of novel applications in fintech, customer support, sports analytics and cyber security. The state-of-art methods in use include neural networks, support vector machines, random forests, decision trees, Adaboost, and others. Mundane tasks have been automated already and more specialised ones are on their way out.
However, the ‘state-of-art’ technologies often encourage a false sense that AI or ML enabled strategies are production-ready. In reality, their deployment usually takes a few months to a few years in a live setup. For instance, in spite of all our advances in natural language processing, we are still a long way from truly intelligent chat bots that can understand and hold a conversation with a human being. Sure, Google had a neat demo of virtual assistance at Google I/O 2018, but the company still has its own breakpoints and faults that are slowly being ironed out.
Michael Jordan, professor of EECS and statistics at Berkeley, claims that we are only beginning to understand the true depth of AI and its potential to impact humankind. Deep learning has produced results that are orders of magnitude better than conventional machine learning algorithms. Deep neural networks have found widespread application in vision, recognition and speech, producing far better results than were seen before.
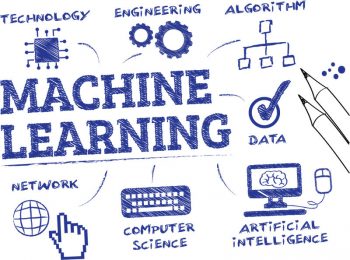
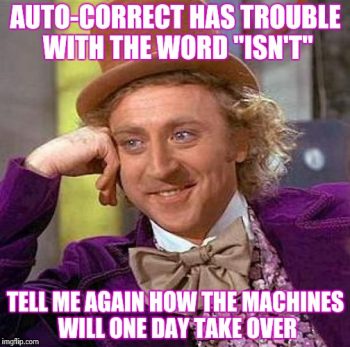
Machine learning applications
Machine learning is being used in various domains that include niche research areas as well as broad segments of customers. It relies on data at its core, since the nature and quantity of data collectively contribute to the performance metrics for the model. Recent focus on deep learning has seen the emergence of convolutional neural networks, recurrent neural networks and long short-term memory as key drivers of R&D efforts in this area. The fundamental sciences have found an analytical toolkit in open source machine learning libraries that are used to code and replicate models.
For fintech, machine learning algorithms drive trading strategies based on game theory and reinforcement learning. In addition, analyses generated by models can evaluate existing and past transactions to detect a possible pattern in investments.
Medical applications vary from detection to diagnosis, but with varying degrees of accuracy. However, digitisation of health records and remote services has indeed made the world a really small place.
Recommendation systems are ubiquitous across e-commerce, video streaming and e-shops. Home automation is gaining traction as virtual assistants improve their learning systems.
Agricultural farms are now monitored and crop diseases detected via machine learning—a project that presents a huge opportunity, especially for agrarian economies such as India. Proof-of-concepts have been set up around Europe already.
Machine learning has thus impacted a far broader set of sectors than just computer science.
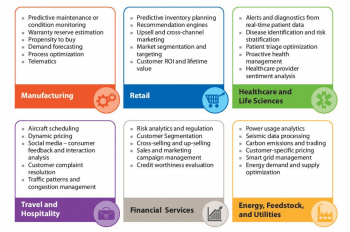
Future technologies
While there is considerable interest surrounding ML and AI, I will attempt to walk you through this territory, leaving out the hype. From a human perspective, it is quite plausible to accidentally create artificial general intelligence that could impact all of humanity. However, we are still years away from such a possible misfortune.
Machine learning has some exciting new developments and future plans. The launch of the ONNX format that allows the interchange of deep neural networks across different libraries, serves as a prelude to the cooperation that is needed to ensure quick, easy and meaningful experiences for all. It is envisioned to take on a central role as research and development of models progresses.
Reinforcement learning is a largely unexplored space that is currently seeing much activity. Examples include deep-q networks, playing Atari and other video games, or learning to walk, run, and jump in a virtual environment.
Truly self-driving vehicles (with Level 5 autonomy), that are still limited to the design desk, should enter the market after being subjected to an extensive set of safety regulations, given the past incidents with Uber, Tesla, Waymo and others.
Quantum AI is a relevant subject area that could take off in the near future, though in its current state it is restricted mainly to theories and hypotheses. It could offer tremendous speed-ups and reinvent the complete pipeline that currently relies on training models on CPUs or GPUs.
Improvements in unsupervised learning may come about as strategies for clustering improve in efficiency. Similar progress will come about in defining the evaluation metrics for clustering that usually relies on assessment of its peers as a group.
Ethics and AI policy come to the fore when you give a company access to all your data, since there is a huge potential for this to be misused. End-to-end encryption, security, anonymity and abstraction of data then gain importance. With the European General Data Protection Regulation rules kicking in, it has forced businesses to rethink their business practices and explicitly seek permission before using our data.
Personalisation will run deeper than usual, with data profiling at the root of the user’s ‘profile’ based on likes, views and browsing patterns.
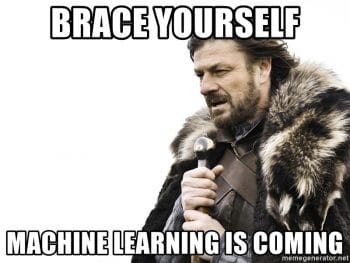
Reproducibility and interpretability are major concerns, especially when treating neural networks as black boxes and seeking to explain the results of experiments, or when attempting to replicate large scale training without the original datasets. Attempting to derive intuitive information about the intermediary steps, learned features and other aspects of the training of a neural network is not easy.
The brain-computer interface will soon be realised, though may be not perfectly. Emulating the constructs underpinning an actual human brain was the original motivation behind developing neural networks; so only the future can tell when this will materialise.
In the years to come, it is possible that we will be able to create some semblance of general intelligence. This can change the world or even destroy it—let’s just keep our fingers crossed that we will all survive!